
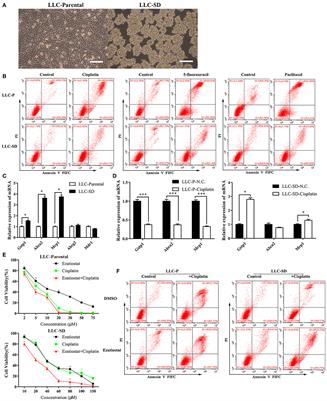
Hypothesis: The distance between windowed dFCs and HE-FC/LE-FC patterns reflects ongoing changes in the task engagement levels, as reflected in variations in short-term task performance (e.g., faster/more accurate responses). Such task-specific high-engagement FC (HE-FC) representative patterns are estimated here using k-means centroids for active tasks. Such a low-engagement FC (LE-FC) representative pattern is obtained here as the k-means centroid associated with resting periods, which serves as a prototype FC pattern for rest.Ĭlaim 3: Task-related whole-brain FC patterns can be regarded as task-specific markers of high engagement/active engagement with a given task. Here we make several claims, which lead to the following testable hypothesis.Ĭlaim 1: Short-term task engagement levels, that is, the amount of mental effort dedicated to the task, are encoded in whole-brain FC dynamics.Ĭlaim 2: The resting whole-brain FC pattern can be regarded as a non-task-specific marker of low engagement/passive engagement during task performance. This approach has recently been shown to be highly replicable (Abrol et al., 2017), predictive of mental illness (Rashid et al., 2016), and correlate with multimodal imaging modalities (Allen, Eichele, Wu, & Calhoun, 2013).Īdditionally, an alternative approach would be to compute representative whole-brain FC patterns that describe how the brain is functionally organized during periods of high and low engagement (HE/LE) during a given task, and use some measure of dissimilarity between windowed dFCs and those HE/LE representative patterns to track short-term engagement level indexed by task performance. The identified FC states were suggested to reflect shifts in ongoing cognition during rest. The pipeline studies the time-varying connectivity between pairs of timecourses coming from independent networks/components, using a combination of spatial independent component analysis (ICA), and k-means clustering of sliding window correlation matrices. ( 2014) proposed a pipeline to investigate whole-brain dynamic FC (dFC) during rest, also called dynamic functional network connectivity (dFNC). Moreover, mounting evidence emphasizes the potential biological and cognitive significance of blood oxygenation level-dependent (BOLD) fMRI FC dynamics evaluated on the brain as a whole (e.g., considering all possible region-to-region connections). Spontaneous fluctuations of FC during rest over short timescales (e.g., seconds to minutes), commonly referred to as FC dynamics (Hutchison et al., 2013), are believed to be primarily driven by neuronal phenomena, as evidenced by studies using simultaneous fMRI and electrophysiological recordings (Chang et al., 2013).
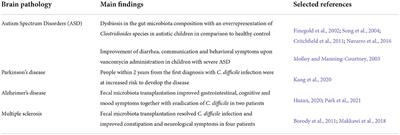
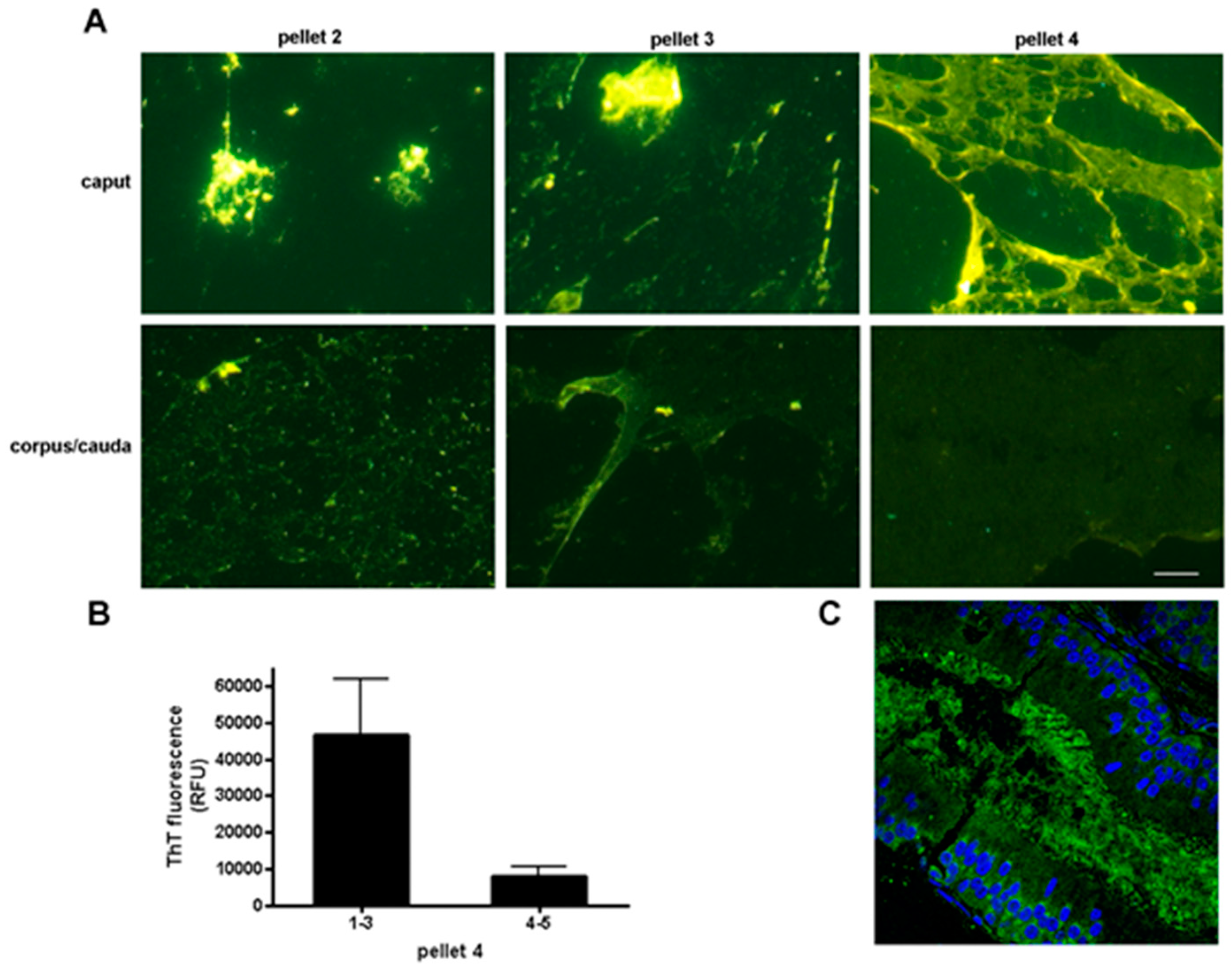
These findings partially confirm our hypothesis and underscore the potential of whole-brain dFC to track short-term task engagement levels.įunctional connectivity (FC) analyses of resting-state functional magnetic resonance imaging (fMRI) data have consistently revealed sets of spatially distributed and temporally correlated brain regions, which correspond to canonical functions such as vision, audition, language, memory, and attention (Smith et al., 2009). Significant relationships were observed between abovementioned metrics and windowed task performance for the working memory task only. Finally, those engagement markers were evaluated against windowed task performance by using a linear mixed effects model. Then, we derived three metrics from whole-brain dFC patterns to track engagement level, that is, dissimilarity between dFC patterns and engagement-specific FC patterns, and the level of brainwide integration level. Next, we extracted two engagement-specific FC patterns representing active engagement and passive engagement by using k-means clustering. First, we estimated dFC patterns by using a sliding window approach. To test this hypothesis, 25 subjects were scanned continuously for 25 min while they performed and transitioned between four different tasks: working memory, visual attention, math, and rest. Here, we hypothesize that dFC patterns carry fine-grained information that allows for tracking short-term task engagement levels (i.e., 10s of seconds long).
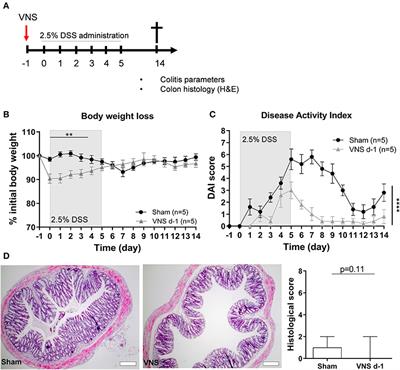
It has recently been found that whole-brain dynamic FC (dFC) patterns contain sufficient information to permit identification of ongoing tasks. Brain functional connectivity (FC), as measured by blood oxygenation level-dependent (BOLD) signal, fluctuates at the scale of 10s of seconds.
